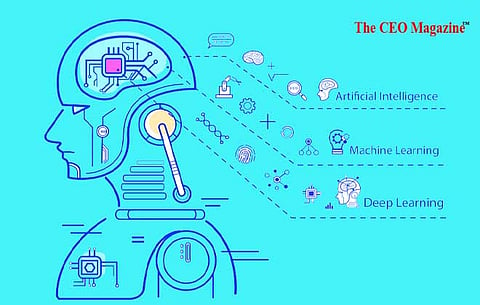
Most In-Demand Tech Skills for Data Scientists
In the rapidly evolving world of technology, staying on top of the latest trends and technologies is important. With so many tools and techniques at your disposal, knowing which ones are worth focusing on can be difficult. According to Glassdoor, Data Science has been the most in-demand skill in the world for quite some time now. Every industry, be it healthcare, real estate, software, or financial services, have great demand for data scientists. Because of the high demand, hiring skilled professionals is a tough task.
There is a shortage of people having the right skills, so organisations must focus on training their existing staff with Data Science skills and keeping their skills up to date with the ever-changing industry. To gain expertise, enrolling in a comprehensive Data Science Course can provide you with the training and knowledge needed to excel in the field.
Certain skills are in higher demand than others, and mastering them can help you stand out in the job market. Following are the skills which you should learn if you want to be a successful Data Scientist.
Python
If you want to explore the field of data science then learning python is important for you as it is known to be the centre to the growth of AI and data science. The programming language is loved by all data scientists, web developers, and AI experts thanks to the simplicity of its syntax.
This language helps to create algorithms and apps more efficiently and its diverse applications across data analysis and AI.
R
The R programming language is mostly used for the statistical analysis of large datasets. It was the preferred tool in the data science community for many years as an ideal resource for plotting and building data visualizations. But the swift performance of python language has made it the first choice for data scientists in the development of AI applications.
Hadoop
Hadoop is open-source software that stores large volumes of data across clusters of computers, which helps organizations to scale and distribute data processes without worrying about the available memory of their computing systems and servers. A data scientist uses Hadoop to quickly process, filter, explore and huge data across numerous clusters.
Machine Learning
Machine learning is a subset of AI that uses data and algorithms to teach computers to identify patterns and take actions or predict those patterns without being purposely programmed to do so.
Machine learning uses structured datasets to train computers a variety of techniques; it is commonly differentiated in many categories like supervised learning, unsupervised learning, and reinforcement learning. Scam detection and recommendation engines are common applications of machine learning.
Natural Language Processing
Natural Language Processing (NLP) is a branch of artificial intelligence that trains computers on reading and understanding language as it's informally used by humans. NLP uses machine learning algorithms to analyze substantial amounts of data on language syntax and semantics, teaching computers to understand human speech inputs and respond accordingly. NLP is at work in digital assistants like Alexa, chatbots for customer service support, and even the legal industry for the quick scan of lengthy legal documents.
Deep Learning
Deep learning is considered as a part of machine learning and uses artificial neural networks, which are computational algorithms, built on multiple data layers. These artificial neural networks are intended to imitate the neural networks of the human brain and learn by observing details in the datasets it's told to study.
Deep learning is used in applications like image recognition in which it trains to recognize group photos of your parents together and also in robotics, teaching robots to recognize common scenarios and to react accordingly in each one.
SQL
SQL (Structured Query Language) is a necessary skill for anyone in a data role and is useful for software engineers and system administrators. SQL is great at data manipulation, allowing teams to run basic to advanced queries and merge data from multiple data sources. Just like python language, it is an important skill to acquire by data scientists
Regression and Classification
Regression and classification are methods of supervised learning where input and output data are provided to the learning algorithms. Both methods attempt to predict a value based on supplied datasets.
Regression algorithms use numerical data while classification algorithms use categorical data. Regression analysis may be used in predicting housing prices based on data of similar houses in similar neighbourhoods. Classification analysis would be used in a mobile app that identifies a plant-based on a user's uploaded photo.
Bottom Line
As the need for artificial intelligence is increasing the requirement for skilled data scientists are also rising. The key to sustaining in this competitive market is to learn from the best Data Science course and have a skilled team of data scientists that can help you achieve success. Once you have built a world-class data science team then you can easily tackle the data challenges within an organization.
Get The CEO Magazine to your Door Steps; Subscribe Now